Emotion Detection using Transfer Learning from Speech and Speaker Recognition Deep Neural Net Models
Joint ASME Westchester Section, SME Chapter 216, and IEEE Tappan Zee Subsection Meeting.
Dr. Homayoon Beigi, President of Recognition Technologies, Inc. and
Adjunct Prof. of Computer Science and Mechanical Engineering, Columbia University
Emotion Detection using Transfer Learning from Speech and Speaker Recognition Deep Neural Net Models
December 2, 2020 7PM – 9PM EST
Virtual Zoom Meeting. You must register to receive the Meeting ID and Passcode.
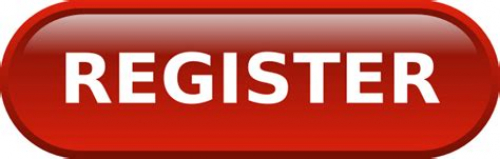
Automated emotion recognition is a challenging task: emotion is abstract, its expression varies across different modalities, the affect of words is context-dependent, and it lacks large datasets. To address these issues, an emotion detection system was built by transfer-learning from speech recognition, speaker recognition, and textual analysis. Some of these models have been built separately and some have already been combined for an improved result. The basic model is based on a multilayer Time-Delay Neural Networks (TDNN) trained on the tasks of speech recognition and speaker recognition using the Tedlium and VoxCeleb corpora respectively. Part of the architecture is then fine-tuned on the task of emotion recognition, using the CremaD corpus. Using this network, emotional speech embeddings are extracted for the CremaD data from different layers of the network and concatenated with text embeddings for the accompanying transcripts using a pretrained model based on Bidirectional Encoder Representation from a Transformer (BERT). The resulting embeddings are used to train a Linear Discriminant Analysis (LDA) transformation followed by a Probabilistic Linear Discriminant Analysis (PLDA) model to produce a scoring metric between two dense embeddings after the LDA process. To understand the merits of each component, an exhaustive evaluation of the predictive power of every permutation was conducted, namely for the TDNN alone, speech embeddings from each layer alone, text embeddings alone, and every combination thereof. Our best variant, trained on only VoxCeleb and CremaD and evaluated on IEMOCAP, achieves an Equal Error Rate (EER) of 38.05%. Including a portion of IEMOCAP during the training improves the 5-fold averaged EER to 25.72% (For comparison, 44.71% of the gold-label annotations by humans include at least one annotator who disagrees). For obtining these results we have only used transfer learning from speaker recognition and textual emotion recognition. Our prior work shows that better (possibly complementary) results may be obtained for emotion recognition based on transfer learning from speech recognition compared to solely transferred from the speaker recognition domain. We are currently pursuing a combination of speaker, speech, and text for an even better performance.
Homayoon Beigi earned his BS, MS, and PhD from Columbia University in 1984, 1985 and 1990 respectively. The author of the first and only comprehensive textbook on Speaker Recognition, for three decades, he has been involved in research and development in Biometrics, Pattern Recognition and Internet-Commerce. He has developed the award-winning RecoMadeEasy® Speaker Recognition and the multiple-award winning, CommerceMadeEasy® software. He has been an Adjunct Professor since 1995, teaching graduate level Speaker, Speech, Handwriting and Applied Signal Recognition, as well as Control Systems courses at the CS, ME, and EE departments of Columbia University and has advised PhD students in the ME and civil engineering departments. He was a Research Staff Member at the IBM T.J. Watson Research Center from 1991 to 2001, working on Speaker Recognition, ASR, LM, Search, Handwriting Recognition, Control, and NN Learning. He developed the SAFE Audio ANSI standard and was an active liaison in the US delegation of the ISO/SC37-JTC1-WG3 and the VoiceXML Forum on Speaker Biometrics. His other research includes Structural Health Prognosis, Image Compression, Kinematics, Financial Optimization, and Zero-Gravity Fluid Dynamics. His "Fundamentals of Speaker Recognition" has been downloaded by more than 70,000 times. His is the recipient of two IEEE best paper awards and 13 issued patents and over 90 peer-reviewed publications. He is an Associate Editor of the AutoSoft Journal, Editor of the BISC Handwriting Recognition, Senior Member of IEEE, on Advisory Board of IEEE Spectrum, and reviewer for the Pattern Recognition Journal, IEEE PAMI, IEEE Transactions on NN, IET Signal Processing Journal, the International Journal of Control, AIAA Journal, ICASSP, Interspeech, and more.
Dr. Homayoon Beigi, President of Recognition Technologies, Inc. and
Adjunct Prof. of Computer Science and Mechanical Engineering, Columbia University
Emotion Detection using Transfer Learning from Speech and Speaker Recognition Deep Neural Net Models
December 2, 2020 7PM – 9PM EST
Virtual Zoom Meeting. You must register to receive the Meeting ID and Passcode.
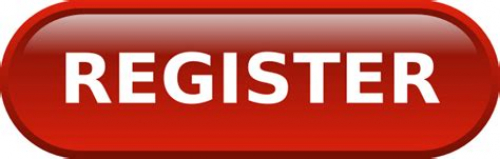
Automated emotion recognition is a challenging task: emotion is abstract, its expression varies across different modalities, the affect of words is context-dependent, and it lacks large datasets. To address these issues, an emotion detection system was built by transfer-learning from speech recognition, speaker recognition, and textual analysis. Some of these models have been built separately and some have already been combined for an improved result. The basic model is based on a multilayer Time-Delay Neural Networks (TDNN) trained on the tasks of speech recognition and speaker recognition using the Tedlium and VoxCeleb corpora respectively. Part of the architecture is then fine-tuned on the task of emotion recognition, using the CremaD corpus. Using this network, emotional speech embeddings are extracted for the CremaD data from different layers of the network and concatenated with text embeddings for the accompanying transcripts using a pretrained model based on Bidirectional Encoder Representation from a Transformer (BERT). The resulting embeddings are used to train a Linear Discriminant Analysis (LDA) transformation followed by a Probabilistic Linear Discriminant Analysis (PLDA) model to produce a scoring metric between two dense embeddings after the LDA process. To understand the merits of each component, an exhaustive evaluation of the predictive power of every permutation was conducted, namely for the TDNN alone, speech embeddings from each layer alone, text embeddings alone, and every combination thereof. Our best variant, trained on only VoxCeleb and CremaD and evaluated on IEMOCAP, achieves an Equal Error Rate (EER) of 38.05%. Including a portion of IEMOCAP during the training improves the 5-fold averaged EER to 25.72% (For comparison, 44.71% of the gold-label annotations by humans include at least one annotator who disagrees). For obtining these results we have only used transfer learning from speaker recognition and textual emotion recognition. Our prior work shows that better (possibly complementary) results may be obtained for emotion recognition based on transfer learning from speech recognition compared to solely transferred from the speaker recognition domain. We are currently pursuing a combination of speaker, speech, and text for an even better performance.
Homayoon Beigi earned his BS, MS, and PhD from Columbia University in 1984, 1985 and 1990 respectively. The author of the first and only comprehensive textbook on Speaker Recognition, for three decades, he has been involved in research and development in Biometrics, Pattern Recognition and Internet-Commerce. He has developed the award-winning RecoMadeEasy® Speaker Recognition and the multiple-award winning, CommerceMadeEasy® software. He has been an Adjunct Professor since 1995, teaching graduate level Speaker, Speech, Handwriting and Applied Signal Recognition, as well as Control Systems courses at the CS, ME, and EE departments of Columbia University and has advised PhD students in the ME and civil engineering departments. He was a Research Staff Member at the IBM T.J. Watson Research Center from 1991 to 2001, working on Speaker Recognition, ASR, LM, Search, Handwriting Recognition, Control, and NN Learning. He developed the SAFE Audio ANSI standard and was an active liaison in the US delegation of the ISO/SC37-JTC1-WG3 and the VoiceXML Forum on Speaker Biometrics. His other research includes Structural Health Prognosis, Image Compression, Kinematics, Financial Optimization, and Zero-Gravity Fluid Dynamics. His "Fundamentals of Speaker Recognition" has been downloaded by more than 70,000 times. His is the recipient of two IEEE best paper awards and 13 issued patents and over 90 peer-reviewed publications. He is an Associate Editor of the AutoSoft Journal, Editor of the BISC Handwriting Recognition, Senior Member of IEEE, on Advisory Board of IEEE Spectrum, and reviewer for the Pattern Recognition Journal, IEEE PAMI, IEEE Transactions on NN, IET Signal Processing Journal, the International Journal of Control, AIAA Journal, ICASSP, Interspeech, and more.
Date & Time
Wednesday December 2nd, 2020 7:00pm EST
End Date & Time
Wednesday December 2nd, 2020 9:00pm EST
Venue
Virtual Zoom Meeting
First Name
Reza
Last Name
Chuback
Email
rc2009@verizon.net
Category Chapter Meeting
Event Map
Event Invitees
1
Going
0
Interested
Event Comments